As we navigate through 2025, Artificial Intelligence (AI) continues to transform industries across the globe. While headlines swing between dystopian job displacement scenarios to utopian dreams of AI-enabled peace and prosperity, the reality of AI’s impact is both more nuanced and practical.
What can be observed is a rush to implement AI solutions. However, in a lot of cases, these organizations have yet to fully identify, yet alone understand, the problem they are solving for. This “solution-first” approach often overlooks the fundamental principle of business: understanding and addressing client needs with a focus on improved business outcomes. However, some companies are taking a more measured, strategic approach to AI integration.
Axioma solutions by SimCorp stands out in this regard – approaching AI with a clear focus on client value. By leveraging their core competencies, rather than trying to replicate the core competencies of their clients, the Axioma team focuses on where AI’s iterative processing power can best enhance productivity. Instead of providing a solution without a problem, the team at Axioma started at the very beginning, analyzing their clients’ workflows and processes, identifying where integrating AI would enhance the productivity of portfolio managers and enable them to add value to the investment process.
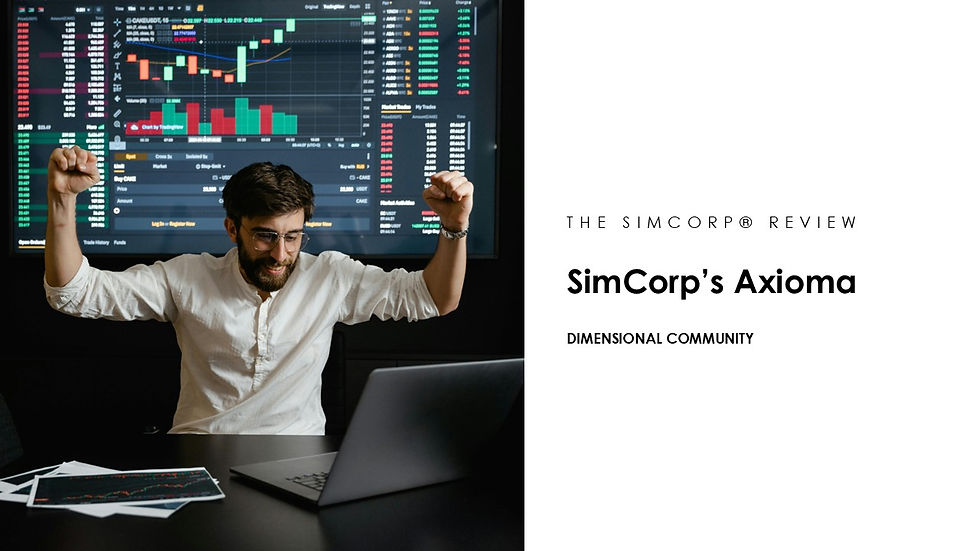
Understanding AI's Role in Investment Finance
If you conducted a poll as to the most likely use of AI in investment finance, alpha generation would be a common response. SimCorp, however, recognizes that this is not their business, it is the business of their clients. So, with the end goal to help their clients maximize alpha generation, SimCorp’s Axioma research and development teams focus their AI efforts to enhance productivity and usability at scale of their existing functional tools.
These functional tools already support the portfolio construction, optimization, and rebalancing workflows at SimCorp's Axioma clients, but managers still need to interpret any simulated results. By leveraging AI to highlight and rank the mandates that managers need to look at, integrating these capabilities into the existing tools will enhance the productivity of portfolio managers, providing greater insight into the investment process. And in these scenarios, the benefits of AI are crystal clear.
Transforming Wealth Management Through AI
Information may be power, but too much information can be overwhelming. In the world of wealth management, where a portfolio manager is responsible for the performance of thousands of mandates, knowing which information to act on is critical.
Assuming your clients are following tax efficient, optimized strategic mandates, one of the daily tasks is monitoring mandates to ensure they stay within target allocations. Those outside the range or ambiguously close to the tolerances need reviewing and determining whether to trade or not is where the portfolio manager exercises their discretion and skill. But when dealing with tens of thousands of mandates, and facing fee compression, efficiency and productivity are crucial, there isn’t time to review every mandate every single day. What managers need are smart tools to help focus where skills are needed.
A portfolio manager is responsible for tens of thousands of mandates. On any given day, if we assume that the need to rebalance those mandates is normally distributed, ranging from those in the left tail that clearly don’t need rebalancing through to mandates in the right tail that do. To maximize efficiency and productivity, what you want is to flatten the curve and push mandates into the tails. You are seeking are fat tails, obvious outcomes that can be automated. The challenge lies in the ambiguous bulk in the middle, where the majority of the mandates sit, requiring review, investigation and decision-making. When the bulk in the middle is too large, it impacts the level of detail you can take any individual mandate review to.
Flattening the Curve with AI-Powered Analytics
What if it was possible to flatten the curve, pushing mandates from the center to the tails where they are either left alone or automatically rebalanced? A tool that learns what managers look at, what they find important, and what they ignore. A tool that learns and understands decision making criteria and uses that to provide a simple ranking score, which can be drilled into for further details, enabling the categorization of mandates into:
the leave alone bucket,
the automatic trading and rebalancing bucket, and
the requires further analysis bucket.
The more managers use the tool, the more it learns and the smarter it gets, benefitting from thousands of mandates running optimizations and simulations each day.
It is such a tool SimCorp's Axioma has built. A single ranking score, referred to as the Axioma WealthLens analytic, lives within the Axioma Wealth Vision functionality. It is built to consume analytics produced for a mandate and quickly learn which analytics managers care about most. The system doesn’t just consume the analytics, it acts on them as well. It understands the optimization strategy for a mandate, incorporating the pre and post optimization statistics, including generated potential trade lists per mandate, and assigns a score (probability) that the portfolio manager would choose to execute the trades. By giving a ranking score with detailed composition analysis available, the system makes life easier for the portfolio manager, who can see a clear priority of which mandates need further review.
Machine Learning and Model Calibration
The mass of mandates involved in wealth management lends itself to the models training. With tens of thousands of data points and observations available daily, the model has a robust weight of inputs to learn from and calibrate itself. If a portfolio manager chooses not to trade a mandate with a high WealthLens analytic score, that feeds back into the model’s calibration, allowing it to learn the soft tradeoffs behind why that mandate wasn’t optimized and traded. These soft trade-offs represent factors that cannot be placed in a mathematical equation. The model constantly learns and calibrates itself to align with the portfolio manager’s thinking. The more it learns, the better its predicted score becomes and the more it can flatten the curve. The ideal target is to leave few to no mandates in the middle, giving the portfolio manager the confidence to automate workflows in the vast majority of cases and concentrate their time, skill, and focus on the few ambiguous cases that require a more nuanced approach.
Institutional Accounts
While wealth management provides abundant data for AI training. Enabling quick model tuning and potentially even less time for a manager to gain confidence in it, this isn’t the case in the Institutional sphere. Managing only a handful of portfolios, often following bespoke strategies, some of which may not even be optimized, presents a different challenge. The type of AI models used for wealth management are not going to learn quickly enough to be useful as it will take too long to obtain the data needed to train it.
This is the issue the Axioma incubation lab is working to solve, how to produce a meaningful analytic with suggested trades to improve a mandate when you don’t know the manager, their strategy, or whether it is optimized. The incubation team started with what they can know, namely history. By analyzing each portfolios’s history, they can determine when it was traded, and its composition reveals what a typical dashboard would have shown the manager in terms of analytics.
Developing a Multi-Variate Model
Taking these basic inputs, the team is developing a multi-variate model based on a neural network. The model incorporates the historical time series of statistics, such as style factor exposures and risk measures, looking for outliers outside of a standard range. There is a binary indicator of whether the portfolio was traded or not, but the labels are low quality because you do not know the reason why, it may have traded for something you are not even tracking, an unknown outlier. It is even possible that the portfolio was not traded even when it should have been, as several outliers could have been flagging but were simply ignored.
The results are a weakly-supervised outlier detection model. The inputs to the model are statistics that flow through the neural network, calculating a binary cross-entropy loss function that looks jointly across all statistics to find the probability (p) that there was an outlier when the portfolio was traded (1) or not (0). By analyzing all the statistics simultaneously rather than one at a time, the model lowers the risk of falsely identifying an outlier. Once you have identified that statistically there is a likely outlier, the system can reverse through the neural network to find the probabilities of which statistic the outlier was. The end result is an alert telling you that you should take action, whilst identifying what led to this decision.
Model Testing and Validation
Testing on this model has shown promising results. Model validation used 30 simulated mandates based on a small set of statistics. On each date, the Axioma Portfolio Optimizer rebalanced each portfolio with a 70% probability if one outlier was present on a given date, and a 95% probability if two or more outliers were present. Each rebalancing returned the sample mandate to the target range of the observed statistics.
After collecting five years of data in the sample mandate – with ongoing efforts to reduce this required timeframe - the model was applied to the sample mandates, including a mixture of statistics that were or were not used in the sample mandate construction. The type 1 error of calling out a false outlier ranged between 2-4%, demonstrating the model’s high accuracy in prompting portfolio managers to trade when the mandate is out of line with its targets. Another smart use of AI to help managers consider items they may not be looking at prompts to initiate trading to bring the mandate back into line.
Optimization Co-pilot
When there is a statistical outlier, the manager needs to rebalance the mandate back to strategic boundaries. There could be various strategies the manager could consider achieving this. Axioma team at SimCorp is utilizing the power of AI to improve the productivity of portfolio managers in this crucial step.
It can take time to specify an optimization strategy. When identifying the outlier, managers often need to act quickly, but navigating the mechanics of specifying the optimization can be time consuming, particularly if there are lots of differing scenarios to investigate. AI allows managers to simply enunciate what they want to do – reduce the tracking error to 1.5% whilst lowering the overweight to large caps to below 100 basis points – instead of building out the full optimization model strategy.
The Axioma optimization API (the same used by portfolio managers for more than two decades now) is exposed to the Large Language Models (LLMs). The LLM then interprets the user’s request and translates it to the functions that need to be called to provide a solution to the user’s request. The LLM is not calculating a portfolio solution itself. Rather, it is calling the same API that the user would call either directly or indirectly through a traditional strategy building GUI. The LLM just “sets” it for the portfolio manager.
A manager can tell Co-pilot what the objectives are and let the AI agent call only the functions needed to complete the task without it surfing the internet for any strange results to throw into the mix. It can then show the manager the new mandate composition with the suggested changes to be made before the manager confirms it, or it can simply ask for more information if not enough detail is available to complete the task.
It couldn’t get any simpler: state what you want to do, review the results, make further enhancements, and when you are ready, send everything off for trading. Everything remains fully transparent, on screen you can not only review the results, but Co-pilot also displays, via the Strategy Builder, the constraints and selections used to build the outcomes. The only downside is that it may do it so fast that there is no time to grab that cup of tea or coffee whilst it’s calculating.
While this doesn’t replace manual optimization strategy building, it can be used for incremental changes, and helps managers make a good start defining a model. Again, it is adding value through AI. Knowing the strategies wanted for rebalancing, executing it, and sending trades to the market remains the value add of the manager, building the actual optimization model to reflect this is not. This allows AI to increase the productivity of the portfolio manager, allowing for more time to be invested where critical thinking is needed.
The Strategic Implementation of AI
We are just scratching the surface of how AI can help our everyday lives. It has an unparalleled power to learn, test, and compute. It is not necessarily coming to take our jobs, but it is coming to try to make those jobs more efficient. By focusing on the parts of their clients’ tasks where time can be burnt for little value added, SimCorp’s Axioma portfolio construction tools are unlocking ways that AI can enhance productivity and free up portfolio managers’ time to concentrate where their skills and intuitions add most value. From pinpointing which mandates need review compared to those that can be automated, through allowing quick build and adoption of optimization models, Axioma tools are empowering AI, and the iterative computing power and learning behind it, utilizing it in a complimentary way to allow managers to focus on where their focus should be. For more details on Axioma solution by SimCorp, check out this webinar.
Whether you're considering adopting AI-powered portfolio management tools or looking to optimize your existing implementation, our team can help you evaluate options, plan your strategy, and ensure successful deployment. Contact us to schedule a consultation and discover how we can help you enhance your investment operations with the latest AI innovations.